Feature Stories
Aaron Dubrow
Related Links
AI Interprets the Mind
New supercomputer-powered techniques are helping researchers understand, diagnose, and decode the brain
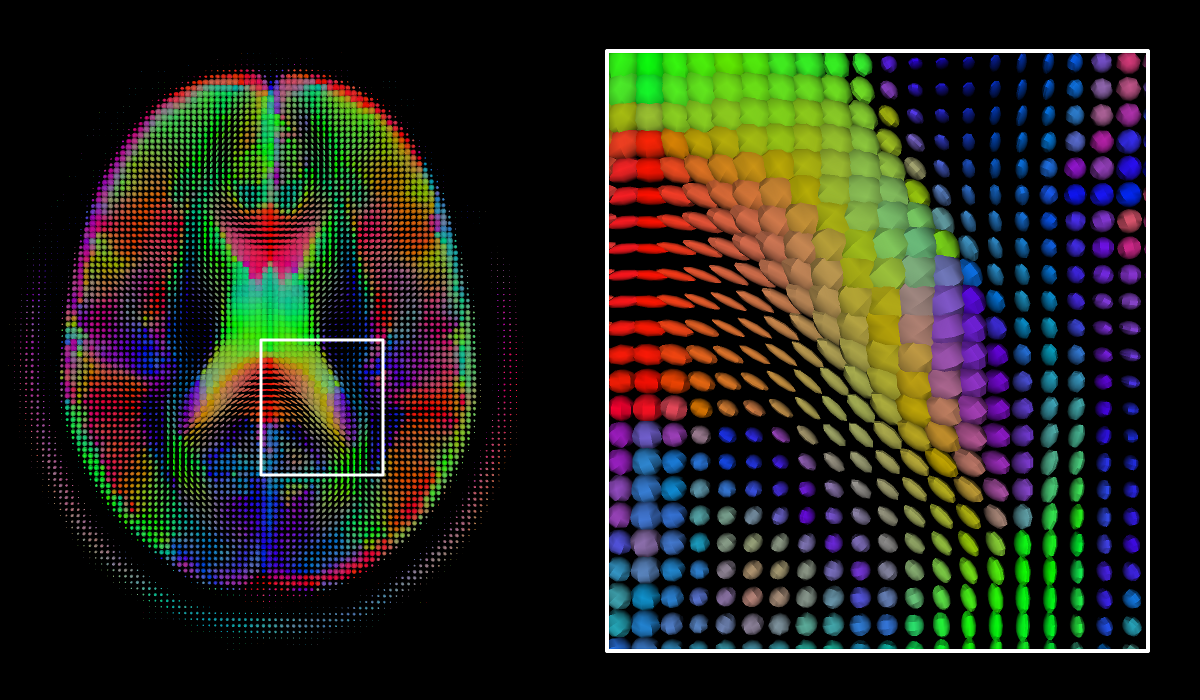
The complexity of the human brain is astounding. With more connections between neurons than stars in the Milky Way, the organ that gives rise to vision, language, and thought is a remarkable product of evolution.
Once, the only way to understand the functioning of the brain was through thought itself. With modern medicine came more thorough anatomical investigations of the parts of the brain. Microscopy brought an awareness of neurons; EEGs helped identify complex signaling patterns among neurons; fMRIs mapped the localization of thought. And newer technologies like positron emission tomography, transmission electron microscopy, and optoelectronics have all added insights into how the brain is structured and operates.
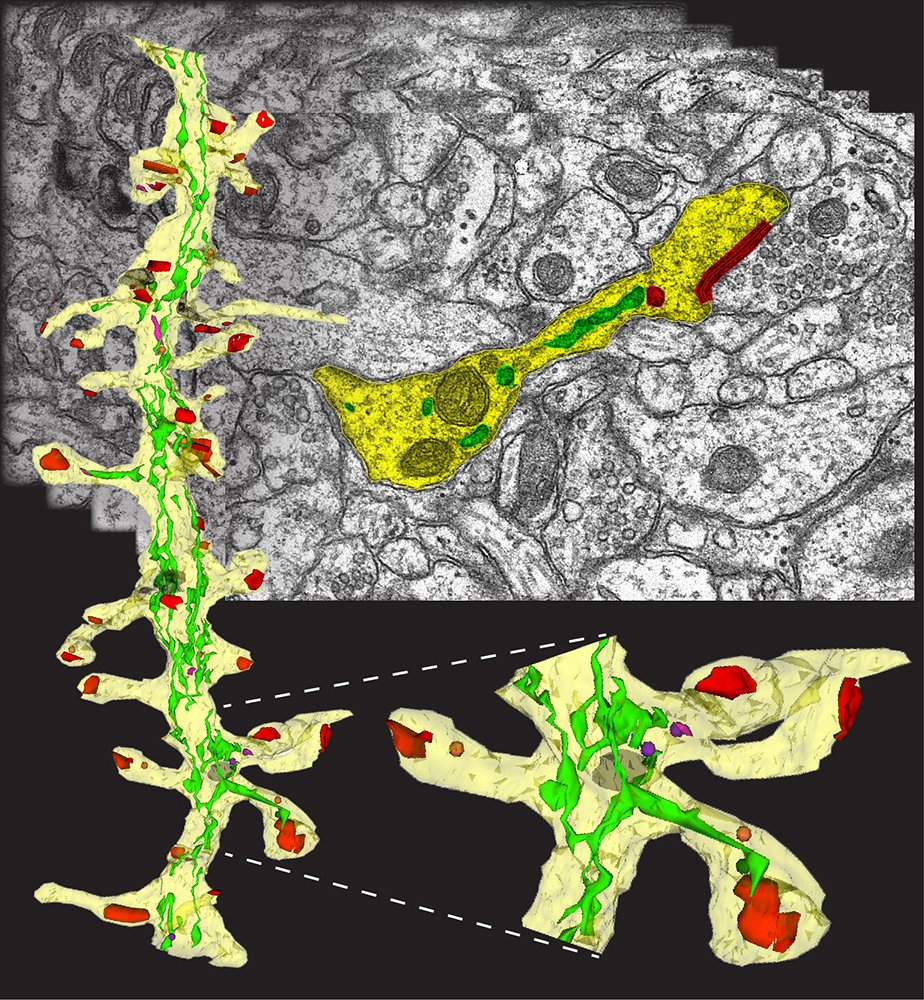
But an important new tool entered neuroscience in the 1970’s and arguably changed the field more than any that had preceded it: the computer.
Advanced computing enables researchers to create detailed 3D models of brain structures, map regions of the brain, and understand the delicate interplay of cells, electricity, and chemicals that enables the brain to understand the world around us and create great works of art.
As computers have grown more powerful over the decades, neuroscientists have applied new techniques to push the boundaries of what can be studied.
Artificial intelligence (AI), in the form of machine learning and deep learning, is the latest such technique to impact the field. AI is advancing computers’ ability to reconstruct images, analyze data, and find meaning in complex patterns of activity, prompting new theories of the mind.
TACC is helping neuroscientists use AI to diagnose diseases, elucidate the function of neurons, and derive fundamental knowledge about the brain.
“The combination of high performance computing, AI, and brain data are powering a revolution in the field,” said Matt Vaughn, TACC’s director of Life Sciences. “It’s opening up a new frontier of knowledge.”
AI feeds on data
Machine learning and deep learning rely on the availability of massive amounts of data to derive models of brain function or ask questions of these models. Scientists can frequently be protective of their data — the raw material for discoveries — but since 2009, Stanford University neuroscientist Russell Poldrack has led an effort to encourage the sharing and pooling of brain data, specifically task-based fMRI data, for the betterment of science.
Originally known as OpenfMRI, the project, with support from TACC and funding from NSF, developed a web-accessible database of more than 500 datasets.
“In recent years, there has been a movement towards the sharing of large, well-curated, and highly general datasets such as the Human Connectome Project,” Poldrack and his collaborator Krzysztof J. Gorgolewski wrote in the science journal, NeuroImage. “The goal of OpenfMRI is to provide a complementary venue for every fMRI researcher to publish their task fMRI data, regardless of the size or generality of the dataset.”
Training AI systems was not necessarily Poldrack’s goal when OpenfMRI launched, but the availability of multiple, large datasets has subsequently allowed researchers, including Poldrack, to begin applying machine and deep learning techniques to their studies.
A team of Japanese researchers recently used OpenfMRI data to develop a deep neural generative model for diagnosing psychiatric disorders; another team used images from OpenfMRI and deep learning methods to develop an MRI quality control tool.
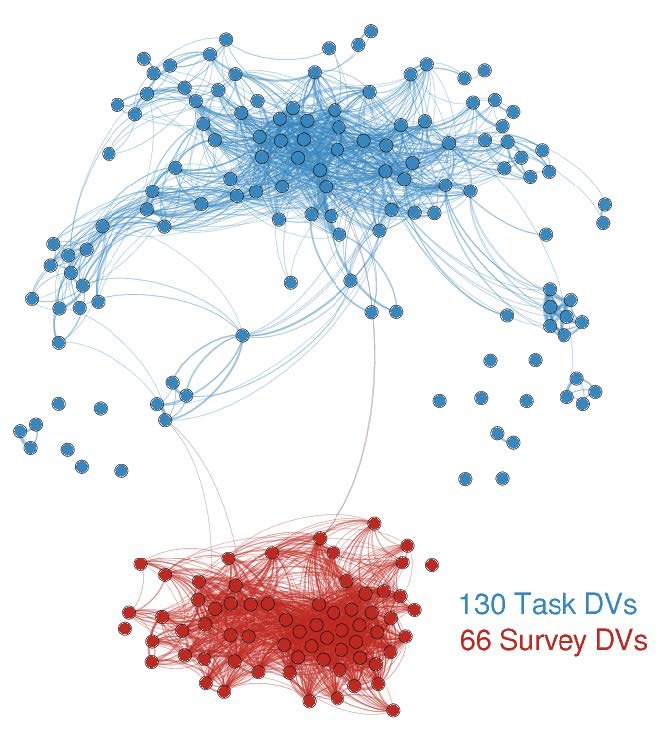
Recently, the project added other modalities of data besides fMRI and was recast as OpenNeuro. Beyond hosting the database, TACC allows researchers to apply machine learning in ways that are not possible elsewhere.
Poldrack’s recent study, published in Nature Communications, used machine learning to explore self-regulation and predict an individual's risk for alcoholism, obesity, and drug abuse based on surveys and task-based studies. They found that by applying more rigorous statistical methods they could uncover underlying structures of the mind, a process they call ‘data-driven ontology discovery.'
"We're bringing to bear serious machine learning methods to determine what's being correlated, and what has generalizable predictive accuracy, using methods that are still fairly new to this area of research," Poldrack said.
For the self-regulation studies, and the more sophisticated deep learning studies he anticipates, advanced computing is critical.
“If you want to build a model of the visual system, there are lots of possible architectures and the only way to know which one is right is to search across all of them,” he said. “You can run one model on a desktop, but for running 10,000 of those models and doing model comparison across them, high performance computing is necessary.”
Applying AI to diseases of the brain
Two researchers using TACC systems and AI to plan brain treatments and diagnose disorders are George Biros and David Schnyer, professors of mechanical engineering and psychology (respectively) at UT Austin.
Biros, a computational expert and two-time winner of the Gordon Bell Prize (a major distinction in the supercomputing world), developed an algorithm that combines mathematical models of tumor growth with machine learning to identify brain tumors in preoperative MRIs with an accuracy that matches that of a trained radiologist.
"Our goal is to take an image and delineate it automatically and identify different types of abnormal tissue,” Biros said. “It's similar to taking a picture of one's family and doing facial recognition to identify each member, but here you do tissue recognition."
Using TACC’s Frontera, the largest supercomputer at any university in the world, Biros is adding many more factors to his machine learning model than was previously possible, and training the systems to detect the extent of cancers beyond the main tumor growth — which is difficult to determine by imaging alone — and to track a tumor to its origin, which can assist in determining the best treatment.
The tissue classifier is being used in clinical trials at the University of Pennsylvania in partnership with his collaborator, Christos Davatzikos, director of the Center for Biomedical Image Computing and Analytics. Predictive simulations guide surgeons to a pre-specified location in the patient’s brain to take tissue from so it can be compared with models to determine their accuracy.
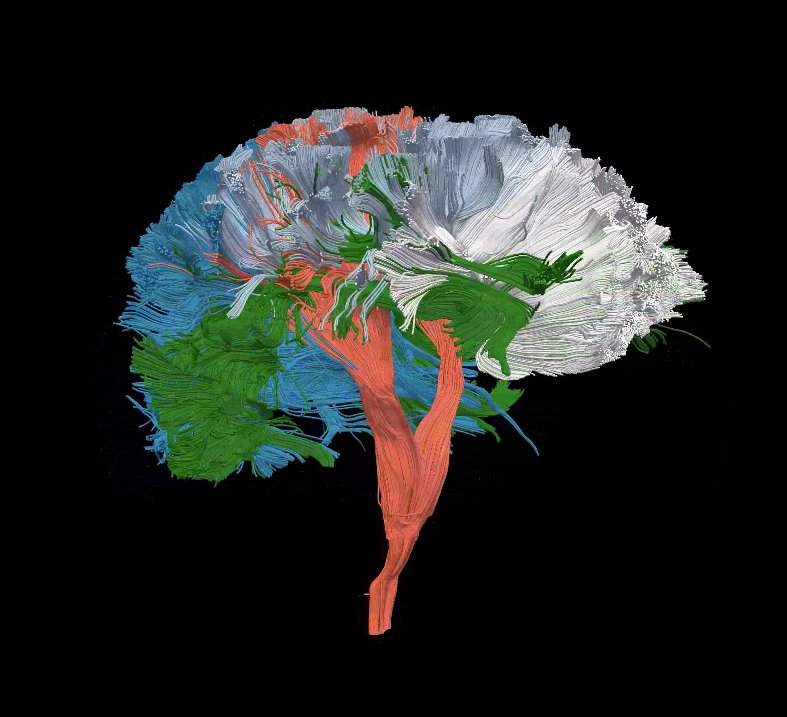
AI-enabled tumor recognition will augment the work of radiologists and surgeons, Biros says, improving the quality of assessments and potentially speeding up diagnosis.
Psychologist David Schnyer has been using similar methods to study aspects of brain function that may signal vulnerability to depression.
His team used machine learning on Stampede2 at TACC to classify individuals with major depressive disorder.
Schnyer and his team labeled features in their brain imaging data that were thought to be meaningful, and marked them as belonging to either healthy individuals or those who have been diagnosed with depression. He used these examples to train the system, and TACC’s supercomputers cycled through the data, fine-tuning a model that could identify subtle connections between disparate parts of the brain. Once trained, the model assigned new subjects to one category or the other. They found it could predict risk for depression with roughly 75 percent accuracy.
“Not only are we learning that we can classify depressed versus non-depressed people using DTI [Diffusion Tensor Imaging], we’re also learning something about how depression is represented within the brain,” said Christopher Beevers, director of the Institute for Mental Health Research at UT Austin and Schnyer’s collaborator. “Rather than trying to find just one area that is disrupted in depression, we’re learning that alterations across a number of networks contribute to the classification of depression.”
Schnyer is currently using machine learning to analyze the results of a three-year, National Institutes of Health-funded study to determine the effectiveness of cognitive therapies for depression, and separately to diagnose age-related cognitive decline and traumatic brain injuries.
“Artificial intelligence has the potential to help us uncover and diagnose diseases much earlier and in ways that were impossible before,” Schnyer said.
Researchers, however, are divided on this application. Poldrack says he is leery of saying deep learning will be useful for diagnosis, per se, but believes AI will help characterize mental health disorders and understand their structure.
“There’s been a lot of debate about the whole way that we talk about disorders,” he said. “Breaking them into diagnostic categories like schizophrenia or depression is not biologically realistic because both genetics and neuroscience show that those disorders have way more overlap than difference. I think that deep learning will help derive new paradigms to understand disorders of the brain.”
Speeding up an 8 trillion year project
For Kristen Harris, a neuroscientist at UT Austin who has spent years studying and modeling a section of the brain smaller than a red blood cell, her efforts to map the brain required her and her team to identify and hand-color structures of the brain in images of brain slices. These were then combined by supercomputers into 3D models that elucidated how the various parts of neurons fit together and enable brain functions.
“Using current methods, it would take eight trillion years of human labor to fully map the connections in the brain,” Harris explained.
"The combination of high performance computing, AI, and brain data are powering a revolution in the field. It’s opening up a new frontier of knowledge."
AI has the potential to help speed up feature identification, structural analysis, and 3D reconstructions of the brain.
“In a billionth of the time that it took me to identify 10 examples of a cell structure in an image, the computer can show me 200 of them,” Harris said. “Every single thing that we discover and can define well, we can delegate to an artificial intelligence system to repeat at scale.”
There’s another way AI can speed up brain imaging: by enabling faster image capture. Recently, Uri Manor, director of the Waitt Advanced Biophotonics Core Facility at the Salk Institute for Biological Studies in San Diego, used TACC resources to create a neural network that can transform low resolution images (which can be captured quickly) into higher resolution ones.
By using pairs of high-resolution and noisy low-resolution versions of microscopy images, they were able to recreate super-resolution images from ones captured at lower resolution. This has the upside of allowing far faster imaging (which in turn allows more coverage of brain areas) and enabling thousands of labs without state-of-the-art equipment to produce images that can resolve small-scale structures in the brain.
“To image the entire brain at full resolution could take over a hundred years,” Manor explained. “But with a 16 times increase in throughput, it perhaps becomes 10 years, which is much more practical.”
What am I thinking now?
A third area of neuroscience research where AI is having an impact is understanding how activities like language processing, vision, and memory formation occur.
Alex Huth, a computer scientist and member of the Center for Theoretical and Computational Neuroscience at UT Austin, uses deep learning and fMRI scanning to localize language concepts and predict, with greater accuracy than ever before, how different areas in the brain respond to specific words.
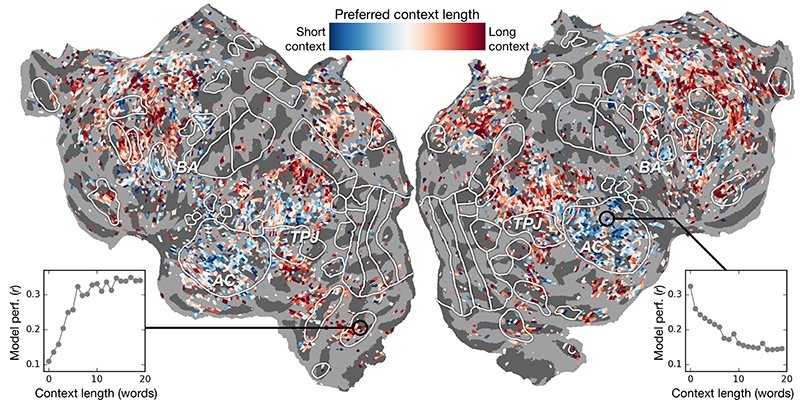
"As words come into our heads, we form ideas of what someone is saying to us, and we want to understand how that happens," said Huth. "Like anything in biology, it's very hard to reduce down to a simple set of equations."
Huth’s work employs a type of recurrent neural network called ‘long short-term memory’ that includes in its calculations the relationships of each word to what came before to better preserve context. The team relied on powerful TACC supercomputers to train the neural network and run a series of computationally-intensive experiments.
These experiments uncovered which parts of the brain were more sensitive to the amount of context included. "There was a really nice correspondence between the hierarchy of the artificial network and the hierarchy of the brain, which we found interesting," Huth said.
This application of AI to brain scans has been used to enable “mind-reading” capabilities for severely-disabled individuals and will continue to advance in the future. “If the technology can be sufficiently miniaturized, we could imagine eventually replacing interfaces that require users to type on a keyboard or speak (like Siri) with direct brain communication.”
Caveats and Opportunities
Though the hype surrounding AI can make the prospect of machines that learn and reason seem inevitable or terrifying, many neuroscientists see limits to the ways today’s machine and deep learning methods can advance neuroscience.
Some, like Thomas Yankeelov, a computational oncologist at UT’s Dell Medical School, fear the field has fallen into a trap with its commitment to ‘Big Data’ and ‘machine learning’ to attack cancer.
“The belief is that if we collect enough data on enough patients, then we will be able to find statistical patterns indicating the best way to treat patients and find cures. This is fundamentally misguided,” Yankeelov recently wrote in an op-ed in The Hill, an online publication that focuses on politics. “Machine learning relies on properties of large groups of people that hide characteristics of the individual patient — this is especially problematic for a disease that manifests itself so differently from person to person.”
Harris, on the other hand, sees benefits from AI systems, such as extrapolating human-labeled data to large corpora that would take hundreds of smart graduate students centuries to sift through, but sees limits to their usefulness as well.
“There's no such thing as AI without intelligence. A human being needs to look at the data from the beginning,” Harris said. “Neuroscience is not just about counting how many synapses are in the brain and determining how they are connected. An AI system won’t ever be able to understand anything new about brain functioning, and there’s still cell biology that needs to be discovered.”
According to Schnyer, the early misuse of machine learning methods led to many poor studies and false conclusions. But the field, as a whole, has learned from its mistakes and moved beyond the simplistic use of machine learning and onto more rigorous studies.
“We’ve learned that we need larger datasets and more sophisticated statistical methods to come to robust conclusions,” Schnyer said. “We also need to strive for greater reproducibility and transparency in the application of machine learning methods.”
This lesson comes with a cost — the need for powerful computers capable of comparing far larger numbers of samples and running many more repetitions to arrive at a solution.
Moreover, in applications like disease diagnosis or treatment planning, the threshold for a trustworthy answer will be high, and resolving issues like the explainability of a deep learning system will be central to building trust.
But the benefits of applying advanced computing and AI to neuroscience are potentially massive. From identifying and slowing cognitive decline, to augmenting human intelligence, to better understanding what it means to love or gain wisdom, the brain is an arena where AI research may offer the greatest rewards.